Artificial Intelligence in Mobility and Transportation: 11 High-Impact Use Cases
At a time when the question is no longer “if” but “how” to deploy Artificial Intelligence, the mobility and public transport sector is transforming into an open-air innovation laboratory. Far from futuristic promises and theoretical concepts, solutions rooted in reality are now essential in the daily lives of transport operators, logisticians and automotive distributors.
We detail 11 impacting initiatives where AI is not a technological gadget, but a real driver of operational transformation. From RATP to Michelin, from Geodis to CarMax, these examples share one thing in common: each shows a measurable and significant ROI, with gains that amount to millions of euros saved, hours freed up and increased customer satisfaction.
Beyond impressive results, these use cases reveal recurring success factors that any organization can replicate. The analysis of these projects makes it possible to identify five determining criteria that have transformed simple experiments into large-scale strategic deployments:
- Anchoring in a clearly identified business irritant — The most successful initiatives respond to operational problems with a real and measurable impact, rather than an abstract desire to “adopt AI.”
- Data quality and accessibility — Organizations or companies that already had structured reference systems enjoyed a significant advantage in deploying and quickly achieving results.
- Early involvement of end users — Projects that integrated field teams from the design phase experienced much smoother adoption and higher utilization rates.
- A gradual and iterative approach — The use cases presented here almost all started with a limited scope, allowing quick adjustments before large-scale deployment.
- Structured change support — Beyond technology, organizations or companies have invested in the training and skills development of teams, making humans the center of transformation rather than their adjustment variable.
Through these sectoral examples, we will see how these principles are applied concretely in contexts as diverse as passenger information, logistics optimization or the customer experience in car dealerships.
Artificial Intelligence and Public Transport: tools to strengthen teams
For Keolis, SNCF and RATP, generative AI is not deployed as a substitute, but as a lever for improving service. The tools make teams more efficient, and relieve them of repetitive tasks in order to make information reliable. Their adoption is systematically based on systems of structured training and integration into business processes.
1. Divide the information time for travelers by 4 — Keolis
Keolis Netherlands has developed an assistant based onGenerative AI, for its internal teams, in order to produce passenger information messages more quickly in the event of a disruption. This system assists operators in generating messages to be broadcast via conventional channels (website, embedded screens, social networks, etc.). As a result, information processing time has been reduced from 20 to less than 5 minutes, with a low initial investment.
At the same time, Keolis has also launched a structured training program to promote the adoption of these tools at the group level. This acculturation plan includes:
- Practical workshops on the drafting of relevant prompts adapted to field uses;
- Raising awareness of the environmental impacts of the use ofGenerative AI ;
- Business pilots to test use cases and capitalize on feedback from the field;
Arnaud Julien, Chief Innovation Officer, insists on the importance of training and mastering the tools:
“We support them to teach them to prompt, that is to say how to make a request to generate reliable and useful answers, avoiding the need to go through it several times, because requests have a carbon footprint.”
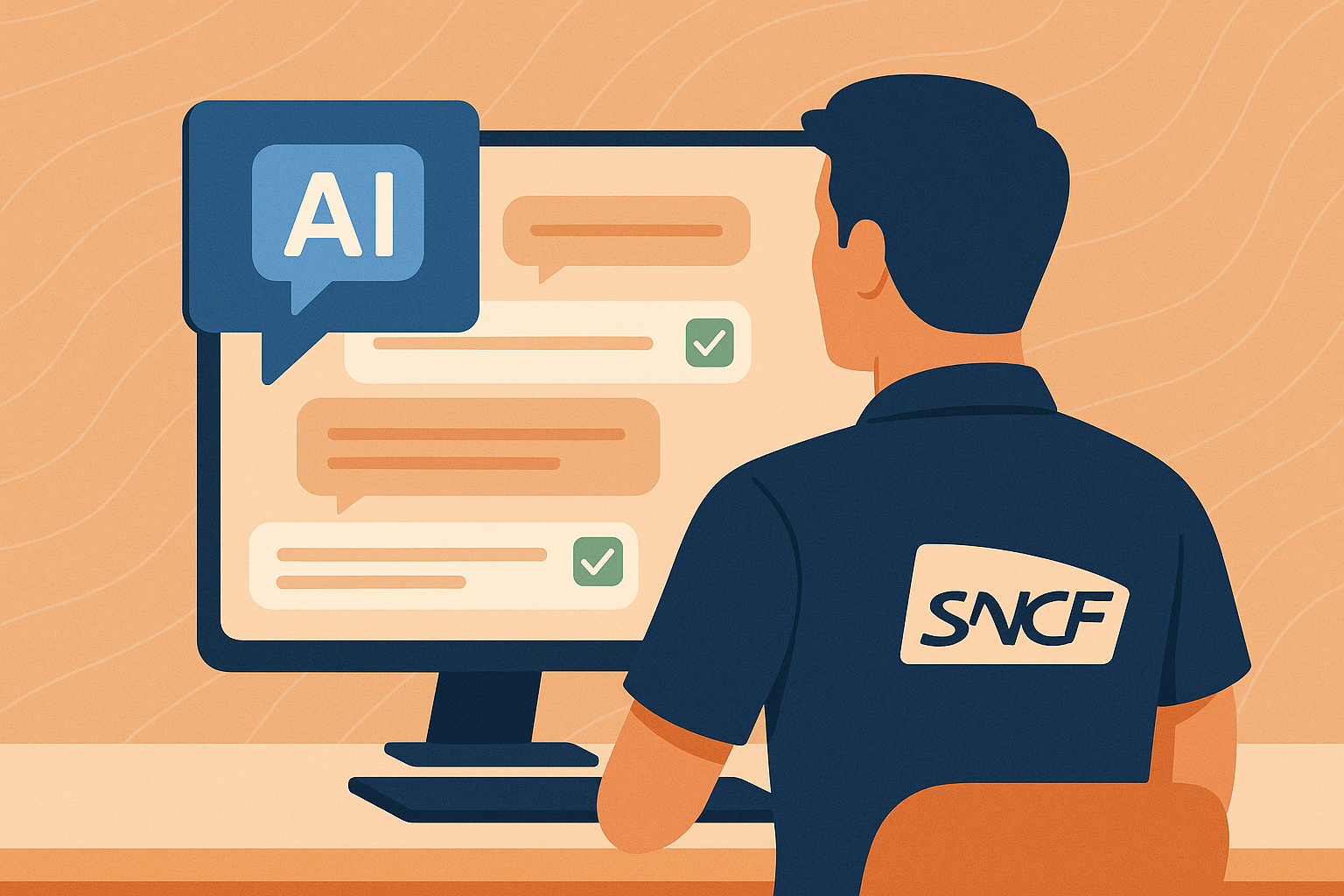
2. At SNCF, 70% of use in messages prepared by an AI assistant
For his part the SNCF deploys several use cases of Artificial Intelligence in its contact centers, aiming to anticipate the volume of requests, to analyze the emotions expressed during the exchanges, and above all to improve the quality of responses. According to Emmanuel Cordente, Chief Technology Officer of SNCF Connect, generative AI makes it possible to pregenerate personalized responses, 70% of which are reused in the final answers, which significantly accelerates productivity while improving customer satisfaction. AI is also used to optimize SEO, content translation, as well as the automated generation of content adapted to search engines.
“We need to be very vigilant about hallucinations. This topic of the veracity of the answers provided via Generative Artificial Intelligence is very important for us. This is why we are further enriching our expertise for use cases' bots', because we must reach a very high level of trust before making them available to our customers.” — Emmanuel Cordente
3. Better productivity and fewer errors: training and equipping agents — RATP
RATP is pursuing a similar approach through its Data Factory, a collective of business and data experts created within the Digital Factory to experiment with real use cases. One of the flagship projects is the conversational assistant CMalin, tested on lines 1 and 3. Dedicated to agents on duty stations, this tool allows them to quickly access complex or rarely used business procedures. It saves significant time, reduces operational errors and promotes versatility.
“The CMalin assistant allows agents to access useful knowledge in situations that they do not encounter on a daily basis. It offers reassurance, reinforces versatility and allows agents to gain efficiency while improving the quality of service offered to travelers and helping to avoid the additional costs associated with the poor application of business actions” — Mathilde Villeneuve, IAgreen Manager, RATP.
This system, designed to be accessible and immediately useful in the field, contributes to a better quality of service and to a reduction in the additional costs associated with bad practices. It illustrates RATP's desire to industrialize the most promising uses of Artificial Intelligence, which has a high value for travelers, agents and territories.
4. Bonus case: -10% of emissions at red lights: Green Light by Google
Here too, AI is used as an assistant to operators of cities that are partners in the Green Light program. Launched by google Research in 2021, he tackles a major urban pollution problem: traffic lights. Urban intersections are responsible for greenhouse gas emissions up to 29 times higher only the roads clear, due to the restart after a stop. By analyzing traffic data from Google Maps, the system identifies simple optimizations for traffic lights (reduction of the duration of red during off-peak hours, synchronization between traffic lights, etc.).
The recommendations generated by the AI are then validated and implemented by municipal engineers — without the need for specific hardware or software. Result: 30 million optimised trips per month, up to 30% reduction in stops and 10% reduction in emissions at some intersections.
“We offer each city dedicated reports with tangible impact metrics, such as how many stops drivers saved at an intersection over time.” — Alon Harris, Program Manager, Green Light
The program is now active in more than 70 intersections across cities such as Rio, Seattle, Bangalore and Boston, and aims for deployments at the scale of Hundreds of cities in the next few years. It reduces fire stops by 30% and pollution by 10%.
Artificial Intelligence & Logistics: towards increased performance
Artificial Intelligence is profoundly transforming the supply chain, bringing measurable performance gains at each stage of the process. From the optimization of tenders to the complete reinvention of the supply chain through simulation, these cases demonstrate that an AI that is well integrated into existing flows quickly becomes a decisive competitive advantage.
5. -80% of time spent on tenders: Artificial Intelligence as a productivity driver — Geodis & DHL
Geodis implemented generative AI capable of automatically writing 80% of tender responses, based on a unified database of past responses, including price elements, historical lines and commercial conditions. This solution made it possible to reduce the time required to process tenders by five, while ensuring better consistency in responses and freeing up time for high value-added tasks. Teams can thus focus on the most differentiating technical or commercial specificities.
“The idea is not to replace teams, but to refocus them on what creates the most value, while responding to all tenders.” — François Bottin, Digital and Technology Director, Geodis
DHL takes a similar approach with a suite of generative AI applications deployed at scale. The first application, used by the Solutions Design team, is used for the cleaning and initial analysis of data submitted by customers. This allows engineers to design logistics solutions more quickly and on a more reliable basis, while reducing time-to-market.
A second application, oriented before sales, supports sales teams from the phase of responding to calls for tenders. It analyzes the needs expressed by customers and automatically generates personalized proposals. This system allows significant time savings, an improvement in the precision of offers and a better distribution of teams' efforts on challenges with higher added value.
DHL also uses GenAI-based business solutions in legal and customer support functions: case summaries, document processing, or request resolution assistance.
“These tools aren't just tech gadgets: they're transforming our key processes.” — Sally Miller, CIO, DHL Supply chain
“By cleaning and analyzing customer data before designing logistics solutions, we increase the productivity of our engineers.” — Markus Voss, Chief Development Officer, DHL Supply Chain
6. +10% capacity, -60% in costs: digital twins are reinventing the supply chain — Michelin & Geodis
In a context of high uncertainty (demand volatility, political and health disruptions), logistics companies are increasingly relying on digital twins to simulate, anticipate and decide. These digital duplicates of the Supply chain allow scenarios to be tested on a large scale by integrating internal data (TMS, WMS, ERP) and external signals (weather, geopolitical hazards, energy prices).
Michelin, supported by Cosmo Tech, has thus led nearly 80,000 simulations to rethink the organization of its production and distribution in Asia. Result: +5% profit, -60% in logistics costs, significant reduction in CO2 emissions. The tool revealed strategies that were very different from those considered by business experts.
“This allowed us to find a way to optimize their supply chain [...] It would have been very difficult to design it without using the tool.” — Michel Morvan, Executive President of Cosmo Tech
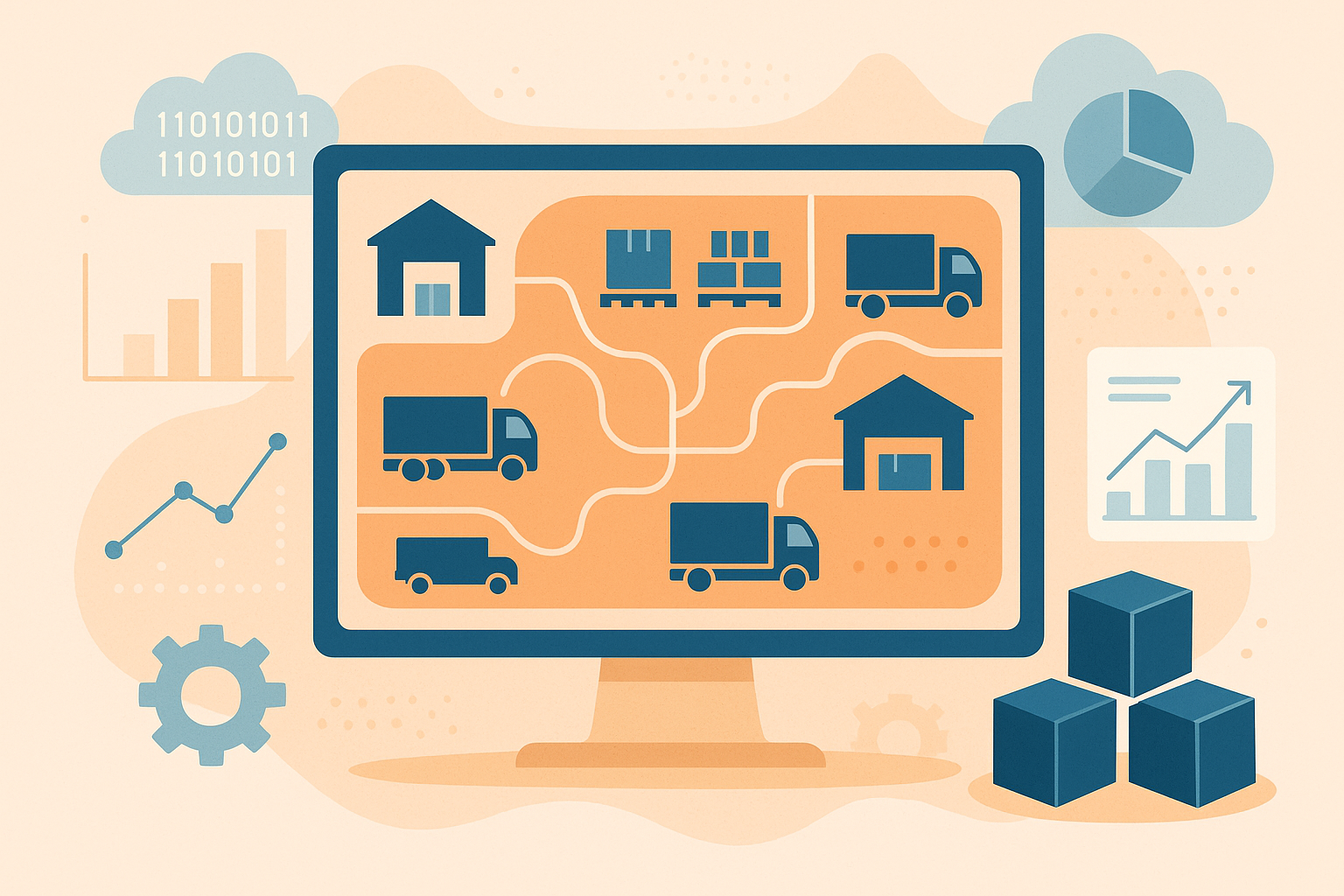
At Geodis, the acquisition of Transoflex in 2022 made it possible to apply these principles to the pharmaceutical last mile. The network has been fully modelled, making it possible to identify logistical overcapacities of 10 to 15%, and to optimize truck loading (+10% packages per tour) via algorithms taking into account manual handling constraints.
“We can now arbitrate between criteria such as CO2 emissions or restrictions in the city center, based on reliable data and a robust simulation engine.” — François Bottin, Digital & Technology Director, Geodis
7. +12% margin and better customer retention: 321 optimizes the last mile
Faced with pressure from a competitor offering more agile logistics and reduced delivery costs, a major retailer called on 321 to rethink its last-mile strategy. The project team, composed of 8 experts (data scientists, developers, mathematicians), was mobilized for six months.
The objective: to develop a yield management algorithm exploiting demand signals, purchase history and price sensitivity to guide customers towards less expensive delivery slots — “Green Slots”. After data analysis and prototyping, a proof of concept was deployed in two logistics hubs.
Results: around 50,000 optimized orders, a 7% reduction in delivery costs (with an estimated potential of 10%), and a margin gain of +12% on strategic products. The project made it possible to better understand customer behavior and to refine pricing policies. It is now deployed in five countries.
“This project shows how good use of data, combined with well-calibrated Artificial Intelligence, can transform a cost center into a profitability lever.” — Romain Ledru-Mathé, Managing Director, 321
Artificial Intelligence and Vehicle Sales: towards an enhanced customer experience
In a rapidly changing automotive market, AI is emerging as a key differentiator in the customer experience and commercial performance of dealerships. From digital touchpoints to telephone interactions, vehicle distributors are rethinking their entire customer journey thanks to intelligent assistants that complement and amplify the know-how of human teams.
8. +11 years gained in content production: CarMax boosts its SEO strategy with Generative Artificial Intelligence/offers
.png)
CarMax, the American leader in the sale of used vehicles, has automated the generation of content for its vehicle search pages using Azure OpenAI Service. Within a few months, the company produced summaries of customer reviews for 5,000 vehicles, a task that would have required 11 years with the traditional manual process. The editorial validation rate reached 80%, guaranteeing alignment with the brand voice.
“With OpenAI Service, we hit that goal in just a few months, and the quality was even better than we anticipated after a little fine tuning.” — Sean Goetz, Director of Application Systems, CarMax
This rich content improves the site's SEO, attracts more traffic, and frees up time for editorial teams, who can focus on high value-added content.
“With the help of OpenAI Service, we're disrupting our industry for a second time by delivering innovative digital tools and capabilities.” — Shamim Mohammad, EVP & CIO, CarMax
9. +30% conversion and 90% of automated requests: Seezar, the dealership co-pilot
Seezar, developed by the startup Seez (UAE), is a sales assistant based on generative AI, specifically designed for car dealerships. Available 24/7, it delivers personalized recommendations, vehicle comparisons and detailed answers to customer questions. Measured results: +30% conversion commercial, 8x faster than a classic chatbot, 90% of requests processed without human intervention.
“Seezar represents a new technological standard for dealerships. By integrating dealer inventory and our years of market data, it offers a smarter way to advise customers.” — Andrew Kabrit, Chief Product Officer, Seez
Launched in the Middle East, the tool is now active in 15 countries, with financial support from Pinewood Technologies Group PLC to accelerate its expansion in the United States. AI is at the heart of Seez's strategy, representing 71% of turnover of the group.
10. From 8% to 100% support: Diago is revolutionizing telephone reception in dealerships
In October 2024, the Citroën dealership in Toulouse-Montaudran only dealt with 8% incoming calls. By integrating the conversational agent of Diago, this rate has increased to 75% human responses, completed by 25% of calls handled by Artificial Intelligence — ensuring complete reachability.
The tool identifies requests, directs calls, or collects the information needed for further follow-up. Billed on a performance-based basis, it allowed a rapid improvement in the quality of service, with a budget that was lower than expected.
“Our customers now benefit from optimal responsiveness, and our teams can focus on their essential missions.” — Laurent Cereda, Director, Citroën Toulouse-Montaudran
Founded at the end of 2023 by Guillaume Couzy, a former Stellantis executive, Diago aims to transform customer service into dealerships using generative AI. The startup signed a deployment agreement with the Peugeot Dealers Group (GCAP).
“Artificial Intelligence is the future of telephone reception in the automotive sector. The tool is efficient and profitable.” — Sylvain Delgendre, Co-founder, Diago
11. +70% customer relationship efficiency: Artificial Intelligence to support field teams — ByMyCar, Hess, Bodemer
These groups rely on AI to streamline exchanges, reduce friction points and increase commercial performance without dehumanizing the customer relationship.
Chez ByMyCar, a conversational AI assistant is developed with Mistral AI and AlloBrain, capable of informing customers about models, stocks, financing or guarantees. By analyzing the group's data (products, VO, financing), it supports customers and alerts teams to sensitive situations in contact centers. This initiative earned BYmyCar the Digital Innovation Prize 2024.
The Hess Automobile Group, elected Customer Service of the Year for the fifth time in a row, makes use of theAI to assist its agents in call centers. The tool allows you to unlock points of friction, while keeping the human being at the heart of the experience.
Finally, Bodemer, which markets 35,000 vehicles per year, invests in Artificial Intelligence to detect the most relevant moments of fleet renewal, by combining usage data, fiscal data and contract types.
“Faced with the heavyweights in the sector, we can only stand out for the quality of the service provided.” — Thibaud Carissimo, Bodemer Group
Conclusion: From experimentation to industrialization
These 11 use cases confirm a fundamental trend: Artificial Intelligence in public transport and mobility is now going beyond the experimental stage to enter a phase of industrialization. The numbers speak for themselves:
- Up to 80% reduction in time spent on repetitive tasks
- Operating margin gains of 10 to 15% on transformed processes
- Sales conversion improvements of 30% and more
The success of these deployments is based on three common pillars:
- A human-centered approach : AI is systematically positioned as a tool for increasing team capacities, never as a substitute. The time freed up is reinvested in tasks with high added value.
- Solid business integration : successful projects are those where Artificial Intelligence is part of existing processes and responds to identified problems in the field.
- Structured support : prompt engineering training, awareness of ethical and environmental challenges, job pilots... The increase in teams' skills is inseparable from technological deployment.
For decision-makers in the sector, the challenge is no longer whether to invest in AI, but to identify the use cases with the greatest impact for their organization. The question then becomes strategic: what are the processes where Artificial Intelligence can create the most value, while strengthening your competitive advantage?
Faced with a market in full consolidation, where players who delay risk seeing their competitiveness erode, it is time for action. The examples presented here show that with a clear vision and controlled execution, the return on investment can be rapid and substantial.
Artificial Intelligence in mobility and public transport is no longer a technology of the future - it is already transforming the present and redrawing the rules of the game for all actors in the ecosystem.
.png)
How to get started with AI in your organization
AI is no longer the preserve of technological giants. Here are five key steps to quickly launch your first impact project:
1. Identify a business irritant with a high potential ROI
- To do : Manage a 2-hour workshop with your field teams to list the 3 most time-consuming and repetitive tasks
- Selection criteria: Prioritize processes with data already available and a quantifiable impact
- Inspiring example: Keolis targeted passenger information, a simple but crucial task
2. Form a business and tech pair
- What to do now: Identify a motivated “business champion” and associate him with a technical profile (internal or external)
- Minimum configuration: A field expert + a developer capable of integrating the APIs of LLMs like Mistral
- Practical advice: Plan 20% of their time dedicated to the project for 8 weeks
3. Get started with existing tools
- Ready-to-use solutions: Exploit language models (OpenAI, Claude, LeChat () via their native interfaces
- Premier cas d'usage: Automation d'un processus de rédaction/analyse répétitif (ex: synthèse de documents, réponses standards)
- Budget starter: 5-10K€ suffisent pour un POC avec les outils SaaS disponibles
4. Fixez un objectif de résultat à 60 jours
- Démarche sprint: Définissez précisément le MVP et ses métriques de succès (temps gagné, erreurs évitées)
- Cadence serrée: Revue hebdomadaire des avancées avec démonstrations concrètes
- Mesure d'impact: Comparez méticuleusement avant/après sur un périmètre limité mais représentatif
5. Industrialisez par itérations
- Post-POC: Élargissez progressivement le périmètre utilisateurs après validation du concept
- Formation pragmatique: Créez un guide simple pour les utilisateurs finaux (max 5 pages)
- Effet boule de neige: Documentez les gains pour convaincre d'autres départements
Cas d'usage starter pour se lancer dès demain:
- Transport public: Assistant de réponses aux réclamations clients
- Logistique: Préparation automatisée des réponses aux appels d'offres standardisés
- Vente de véhicules: Générateur de fiches produits et descriptions commerciales
La clé du succès réside dans le choix d'un premier projet suffisamment simple pour être livré rapidement, mais assez impactant pour créer un effet de démonstration irrésistible dans votre organisation.
.png)